Diabetes is epidemic and many diabetics lose their vision. Diabetic retinopathy is treatable, so it makes sense to screen for it. But screening doesn’t happen as much as it should, in part because there aren’t enough people who can do it. So it would be great to have an inexpensive and accurate screening procedure for diabetic retinopathy.
In JAMA, Varun Gulshan and colleagues report on a neural network that can identify diabetic retinopathy from retinal images. They used a deep learning algorithm that taught itself to identify retinopathy by analyzing a lot of images that had been previously classified as cases or non-cases by experts. Then they tested the algorithm on fresh images and found that it accurately identified retinopathy. This may be the beginning of an important change in medicine.
Objective To apply deep learning to create an algorithm for automated detection of diabetic retinopathy and diabetic macular edema in retinal fundus photographs.
Design and Setting A specific type of neural network optimized for image classification called a deep convolutional neural network was trained using a retrospective development data set of 128 175 retinal images, which were graded 3 to 7 times for diabetic retinopathy, diabetic macular edema, and image gradability by a panel of 54 US licensed ophthalmologists and ophthalmology senior residents between May and December 2015. The resultant algorithm was validated in January and February 2016 using 2 separate data sets, both graded by at least 7 US board-certified ophthalmologists with high intragrader consistency.
Exposure Deep learning–trained algorithm.
Main Outcomes and Measures The sensitivity and specificity of the algorithm for detecting referable diabetic retinopathy (RDR), defined as moderate and worse diabetic retinopathy, referable diabetic macular edema, or both, were generated based on the reference standard of the majority decision of the ophthalmologist panel…
Results* The EyePACS-1 data set consisted of 9963 images from 4997 patients…; (prevalence of RDR = 7.8%); the Messidor-2 data set had 1748 images from 874 patients (prevalence of RDR = 14.6%]). For detecting RDR, the algorithm had an area under the receiver operating curve [AUC] of 0.991 (95% CI, 0.988-0.993) for EyePACS-1 and 0.990 (95% CI, 0.986-0.995) for Messidor-2. Using the first operating cut point with high specificity, for EyePACS-1, the sensitivity was 90.3% (95% CI, 87.5%-92.7%) and the specificity was 98.1% (95% CI, 97.8%-98.5%). For Messidor-2, the sensitivity was 87.0% (95% CI, 81.1%-91.0%) and the specificity was 98.5% (95% CI, 97.7%-99.1%). Using a second operating point with high sensitivity in the development set, for EyePACS-1 the sensitivity was 97.5% and specificity was 93.4% and for Messidor-2 the sensitivity was 96.1% and specificity was 93.9%.
How good are these results? I’ve done a lot of work developing screening instruments for mental health. We never get AUCs this close to 1.0.
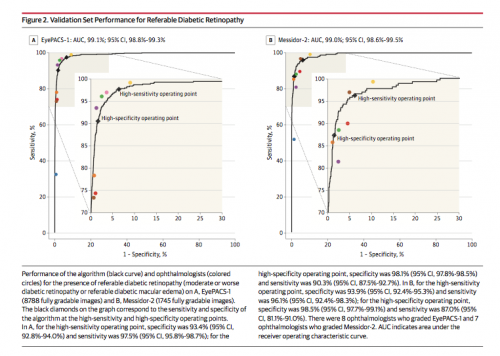
A recent Cochrane Review summarized evidence for another method for detecting diabetic macular edema (one of the conditions Gulshan et al. screened for) and found that
In nine studies providing data on CSMO (759 participants, 1303 eyes), pooled sensitivity was 0.78 (95% confidence interval (CI) 0.72 to 0.83) and specificity was 0.86 (95% CI 0.76 to 0.93).
Gulshan et al.’s neural network was substantially more accurate than that. However, the Results do not mean that the neural network is ready for deployment. JAMA published several commentaries, by people better informed than me, which discuss reasons why this technology may need further evaluation.
Let’s stipulate, however, that screening for diabetic retinopathy by a machine learning algorithm is going to work. (And if it does, it’s likely that machine learning algorithms will also succeed at other important diagnostic tasks.) What will that mean for medicine?
First, the machine learners will screen better than humans do. The authors argue that
This automated system for the detection of diabetic retinopathy offers several advantages, including consistency of interpretation (because a machine will make the same prediction on a specific image every time), high sensitivity and specificity, and near instantaneous reporting of results.
What happens to total health care spending? Automation makes things cheaper, right? Beam and Kohane observe that
Once a [neural network] has been “trained,” it can be deployed on a relatively modest budget. Deep learning uses a… graphics processing unit costing approximately $1000… [that] can process about 3000 images per second… This translates to an image processing capacity of almost 260 million images per day (because these devices can work around the clock), all for the cost of approximately $1000.
Does this mean that screening by neural network will reduce health care spending? That’s unlikely. If the price of an accurate screen for diabetic retinopathy falls, we will screen more. If we screen more, we’ll uncover lots of retinopathy. Finding more people who can’t see will increase the demand for expensive ophthalmalogic treatments. Therefore, unless we also find a way to make those treatments less costly — or find a cheap way to prevent diabetes — automated screening will likely increase total health care spending.
That’s not necessarily a bad thing. I’d rather have a world in which we spend more on health care but many fewer people lose their vision.
And what happens to radiologists in a world of cheap, quick, and accurate neural network screeners? Saurabh Jha and Eric Topol think radiologists won’t necessarily disappear.
The primary purpose of radiologists is the provision of medical information; the image is only a means to information. Radiologists are more aptly considered “information specialists” specializing in medical imaging.
What needs to be added to a screening result to transform it into medical information? One thing is an interpretation of the clinical significance of the finding. Right now, radiologists (and other physicians) can make these interpretations, while computers cannot. So if screening gets cheap and becomes more common, the demand for interpretation increases. Clinicians therefore remain in demand, so long as they are able to add value to the screening result.
Which is to say, until machines also learn to judge the clinical significance of findings better than physicians can.
Finally, automated screening could also improve access to global health care. According to WHO,
the number of adults living with diabetes has almost quadrupled since 1980 to 422 million adults. This dramatic rise is largely due to the rise in type 2 diabetes and factors driving it include overweight and obesity.
Moreover, these factors driving the diabetes epidemic are increasing. But 85% of the world makes $20 USD / day or less. There is no serious near-term prospect of deploying physicians to screen the vision of most of humanity. However, artificially-intelligent systems served by less expensive staff could be deployed globally.** Thus, machine learning could screen many populations that currently have little or no access to specialized care.
The catch, of course, is that it would be pointless to screen the other 85% unless we were prepared to also offer them treatment. The development of cheap, quick, and accurate will confront us with the question of whether we believe in one global standard of health care.
*To interpret the Results it is helpful to know that:
- Sensitivity = Proportion of Cases Correctly Identified (range 0.0 to 1.0).
- Specificity = Proportion of Non-Cases Correctly Identified (range 0.0 to 1.0).
- Area Under the Receiver Operating Curve [AUC] is a statistic that summarizes information about Sensitivity and Specificity (range 0.5 to 1.0).
**Machine learners get better with practice. Deploying the neural network globally would accelerate the rate at which they learn by giving them access to many more images.