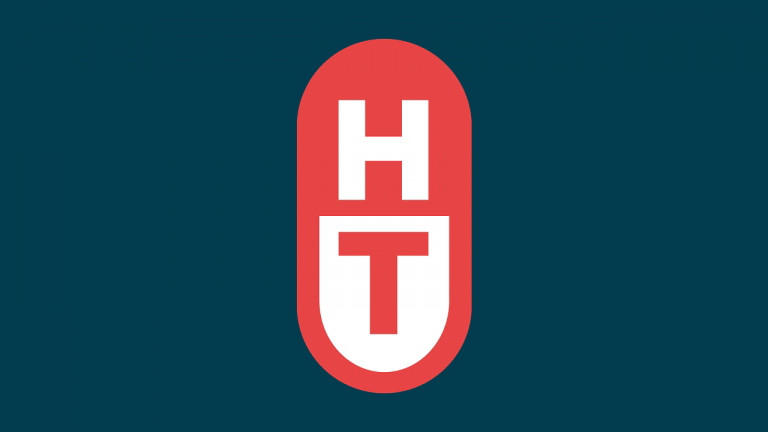
New Obesity Drugs May Impact Mental Health
In mid to late 2023 there was a flurry of news reports about patients taking new weight loss drugs reporting associated mental health concerns, including
In mid to late 2023 there was a flurry of news reports about patients taking new weight loss drugs reporting associated mental health concerns, including
In mid to late 2023 there was a flurry of news reports about patients taking new weight loss drugs reporting associated mental health concerns, including
Rural Veterans face more barriers to health care than those living in urban areas, and the challenges are even greater for those receiving long-term supports and aging services.
If you or someone you love has a life-threatening food allergy, you have to remain constantly vigilant for even the slightest exposure to that food,
Measles is really contagious and can easily spread in pockets of unvaccinated people. In February 2024 a health advisory was issued by the Florida Department
A PEPReC policy brief discusses the impact of a web-based dashboard (STORM) that reviews Veterans’ risk of experiencing opioid-related adverse events.
This brief shares PEPReC’s DEIJ evaluation findings and explains policy changes that could improve access to care and mortality risk factors for Veterans with marginalized identities.
Primary care providers play a key role in assessing cognition and managing care for patients with dementia, but remain undertrained and unequipped to deal with this crisis.
The American healthcare debate is often a pendulum swinging between two extremes: maintaining the status quo and adopting a single-payer system. But what if we’re
Submission deadline for abstracts: Monday 17 June 2024
A recent study sheds light on how shifting economic tides impact quality of care in Veterans Health Administration.
We love some good vaccine data, and we were pretty excited to see a new, long-term study published this month on cervical cancer outcomes after
According to a recent study, “Oreo Cookie Treatment” is better at lowering LDL cholesterol (the “bad” cholesterol) than high-intensity therapy with cholesterol-lowering drugs called statins.
Work posted here under copyright © of the authors • Details on the Site Policies page